Objective
Assess remaining reservoir volumes and identify potential infill targets
Solution
Combine machine learning algorithms with reservoir modelling and the knowledge of the subsurface team in one streamlined process
Outcomes
Improved understanding of sand distribution and intra-compartment communication
• Reduced turnaround time with combined modelling solution
• Identified three infill locations of similar economic interest
• The results suggest these should be drilled earlier than initially planned for optimal NPV
Project Description
Machine learning in context
Machine learning has been in use for many years in the petroleum industry, e.g. kriging and decline curve analysis. Increases in computational power have encouraged further development of algorithms. One focus has been with purely data driven algorithms in big data with production analytics, especially in unconventional plays. Another has been with model-driven machine learning algorithms. This has proven to be a valuable approach since data is combined with underlying physical behavior through models. In the area of reservoir modelling and data conditioning, this application is especially useful when dealing with limited data, large uncertainty ranges and critical business decisions.
Model-driven algorithms
Traditionally, reservoir modelling and dynamic data conditioning have been done in a step-wise, manual or semi-automated manner, making it difficult and time consuming. With the combination of the right algorithms and repeatable modelling workflows using ResX, it was possible to automate this process while accounting for all static and dynamic data in a consistent manner across the modelling chain. Adding to this the combined expertise of the subsurface team led to an improved understanding of the field.
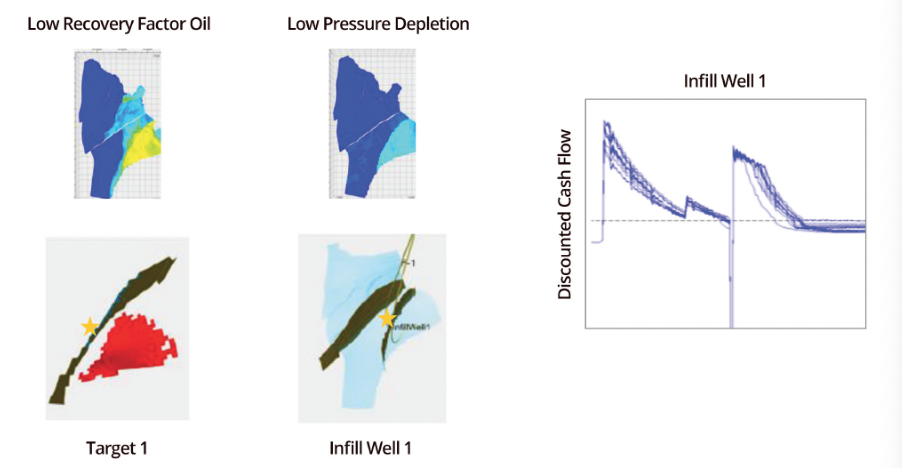
Solution Highlights
32 million uncertainty parameters were used in the model calibration process.
• The data calibration process detected when there were critical issues in the input model parameters and corrected the model parameters to reduce the mismatch, all while the static data information and the geological concept were preserved in the posterior ensemble of models.
• Using the ensemble of data-conditioned reservoir models as input, the prescriptive analytics solution was applied to:
1) Estimate the probability of finding large connected volumes of good sand (above the contact), connected volumes of remaining oil, and connected volumes with little pressure depletion;
2) Identify the intersection between these volumes where the probabilities were larger than a given threshold;
3) Calculate the largest connected volumes of these intersections;
4) Automatically create infill wells within the identified targets, taking physical constraints such as dogleg severity into account;
5) Run predictions for the ensemble of model realizations including the new well targets and calculate the discounted cash flow for all cases.
• All infill well scenarios were economical with no significant difference; however, each case showed negative cash flow earlier than expected which suggested that the infill strategy should be accelerated for optimal NPV.
Asset highlights
The Gjoa field, located off the coast of Norway at the height of Florø was discovered in 1989. Gas occurs above the oil zone in the Viking, Brent and Dunlin groups, in a number of tilted fault blocs. Reservoir porosity and permeability is variable and fault connectivity is unknown.
Planning to put the field in production started in 2006, and it came online in 2010, with the gas exported to Scotland and the oil to Bergen through existing infrastructure.
References
For more details see SPE paper 188557
Gjøa Field, North Sea Offshore Norway, image courtesy of Neptune Energy